Think: Long-Horizon Dynamics
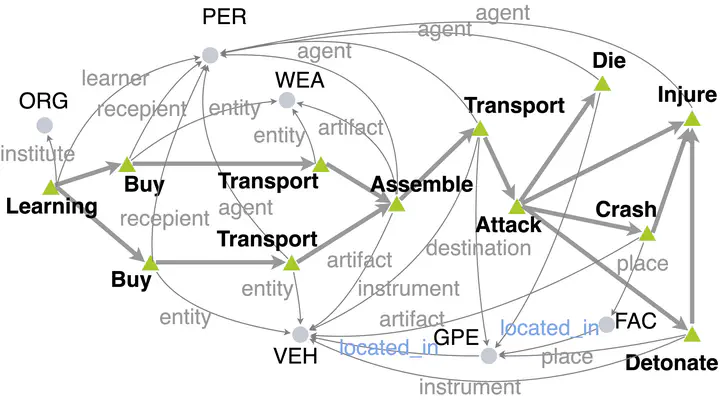
Event extraction from massive multimedia data enables us to obtain a large number of historical events. These historical events imply knowledge about event interactions, which guides our predictions as to what might happen next and what events are missing. For example, after an ATTACK event there will usually be ARREST and SENTENCE events. We refer to this knowledge as Event Schemas, which can be viewed as “complex event templates” that encode knowledge of stereotypical event structures and show the progression of event evolution. However, previous event schema induction work has been oversimplified to be local and sequential, rendering them incapable of dealing with real-world events with numerous participants, complicated timelines, and various alternative outcomes.
My research tackles this issue with a new paradigm of event schema knowledge: an Event Graph Schema, which is a graph-based schema representation that encompasses events, arguments, temporal connections and argument relations. Figure 1 shows an example schema of the complex event type car-bombing: a person learns to make bombs, purchasing materials and a vehicle; a bomb is fixed to the vehicle; and the attacker drives the vehicle to attack people. In this scenario, people can be hurt by the vehicle or by the bomb’s explosion. It is the first application of graph generation to induce event schemas and predict future events.
My work is a new step towards the semantic understanding of inter-event connections. Different from traditional methods using one-hop relations as connections between events, I learn a complicated graph including temporal dynamics and multiple paths involving entities (coreferential or related arguments) that play important roles in a coherent story. Compared to traditional schemas, my new paradigm of Models as Schemas add predictive power to produce multiple hypotheses with probabilities, along with structural justifications for participant-specific and attribute-specific connections.