Write: Truthfully
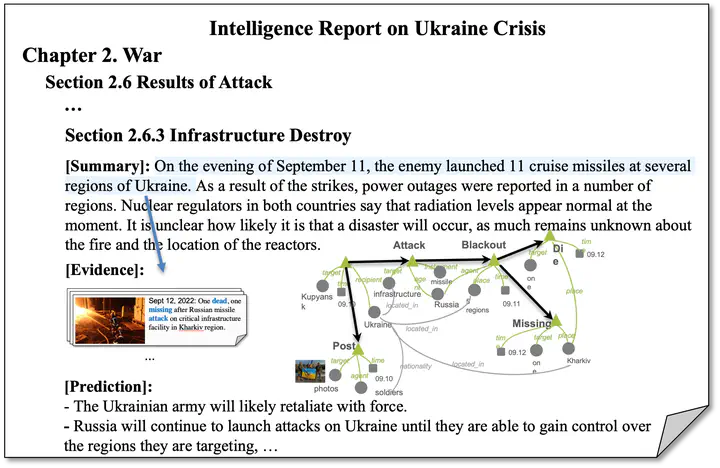
One of the main bottlenecks of large corpora analysis is the multi-document encoding. Whereas existing studies have built text graphs by augmenting text sequences with different hidden structural information, they are typically entity-centric and overlook the events’ intra-structures (arguments) and inter-structures (event-event connections).
My solution is using event graphs to provide a new comprehensive representation and necessary inductive bias. I propose to define the multi-document joint representation as the contextualized embeddings of the nodes on the event graph and collectively model events and arguments. These event graphs can then be used to address the massive unstructured data challenge in real-world applications: (1) Timeline Summarization is formulated as an event graph compression problem and then I design time-aware optimal transport to obtain the summary graph. (2) Meeting Summarization leverages agenda-based topics to segment meeting transcripts, and takes advantage of multi-modal sensing of the meeting environment, such as cameras to capture each participant’s head pose and eye gaze. (3) Multimedia News Question Answering employs multimedia event graphs to condition synthetic question-answer generation, and to automatically augment data via weak supervision.
My work is the first study to use event graph representations to overcome fundamental challenges in handling massive unstructured data that exist in various applications. It provides tangible guidelines to use event structural knowledge in practice, and shows positive results on long-standing open problems in event tracking